Algorithmic Attribution
Algorithmic attribution is a data-driven attribution model that leverages machine learning and statistical analysis to assess the impact of various marketing touchpoints on a customer’s journey toward conversion. By analyzing complex data, algorithmic attribution provides marketers with highly accurate insights into which marketing activities drive results, making it one of the most precise and advanced methods of attribution in digital marketing.
How Algorithmic Attribution Works:
Unlike rule-based models, which assign pre-set values to each touchpoint, algorithmic attribution dynamically allocates credit based on the observed influence of each interaction within the customer journey. Using predictive analytics, algorithmic models analyze historical data to determine the contribution of each touchpoint, adjusting values based on patterns in customer behavior.
Key Benefits of Algorithmic Attribution:
- Informed Budget Allocation
By identifying high-impact touchpoints, algorithmic attribution enables companies to allocate budgets more effectively, focusing on channels that deliver the highest returns. - Bid Optimization
With algorithmic attribution, marketers can optimize bids for search and display ads based on touchpoint performance, increasing the efficiency and ROI of digital ad spend. - Personalized Messaging
Insights from algorithmic attribution enable businesses to tailor messages based on the touchpoints that resonate most with different audience segments, enhancing campaign relevance and engagement.
How Algorithmic Attribution Models Are Built:
Data scientists typically design and maintain algorithmic attribution models. These models require substantial data for accurate predictions, meaning companies must have robust tracking and data collection in place. The algorithms analyze historical customer journeys, assigning value to each interaction based on its probability of driving conversions.
How Algorithmic Attribution Differs from Other Attribution Models:
- Rule-Based Models
Unlike rule-based models like first-touch or last-touch attribution, algorithmic attribution adapts to real-world customer behavior, assigning credit dynamically based on the effectiveness of each touchpoint. - Data-Driven Insights
Algorithmic models use vast amounts of data, providing a more nuanced understanding of the customer journey by identifying patterns that wouldn’t be visible in simpler models. - Customization for Touchpoints
Algorithmic attribution enables marketers to create custom algorithms for specific business needs, allowing brands to adapt to the unique role each touchpoint plays in the conversion process.
Use Cases of Algorithmic Attribution:
- Optimizing Multi-Channel Campaigns
Algorithmic attribution helps brands determine which channels are contributing the most to conversions. This insight can lead to a more balanced strategy that optimizes for both high-performing and emerging channels. - Improving Conversion Rates
By accurately tracking each touchpoint’s contribution, marketers can focus on the interactions that drive the most conversions, increasing overall conversion rates and ROI. - Understanding Customer Behavior
Algorithmic models reveal detailed insights into customer behavior, showing how different touchpoints influence the decision-making process and enabling brands to adjust campaigns for maximum impact.
Challenges and Considerations:
- Data Quality
Algorithmic attribution relies on high-quality, comprehensive data. Incomplete or inaccurate data can reduce model accuracy, so maintaining precise data collection practices is essential. - Complexity and Cost
Building and maintaining algorithmic attribution models requires advanced data science skills and often comes with significant costs, making them more suitable for businesses with larger budgets. - Cross-Channel Integration
To accurately assign value across all touchpoints, brands need a cohesive, cross-channel tracking system. Ensuring that all marketing channels are integrated can be a technical challenge but is crucial for effective attribution.
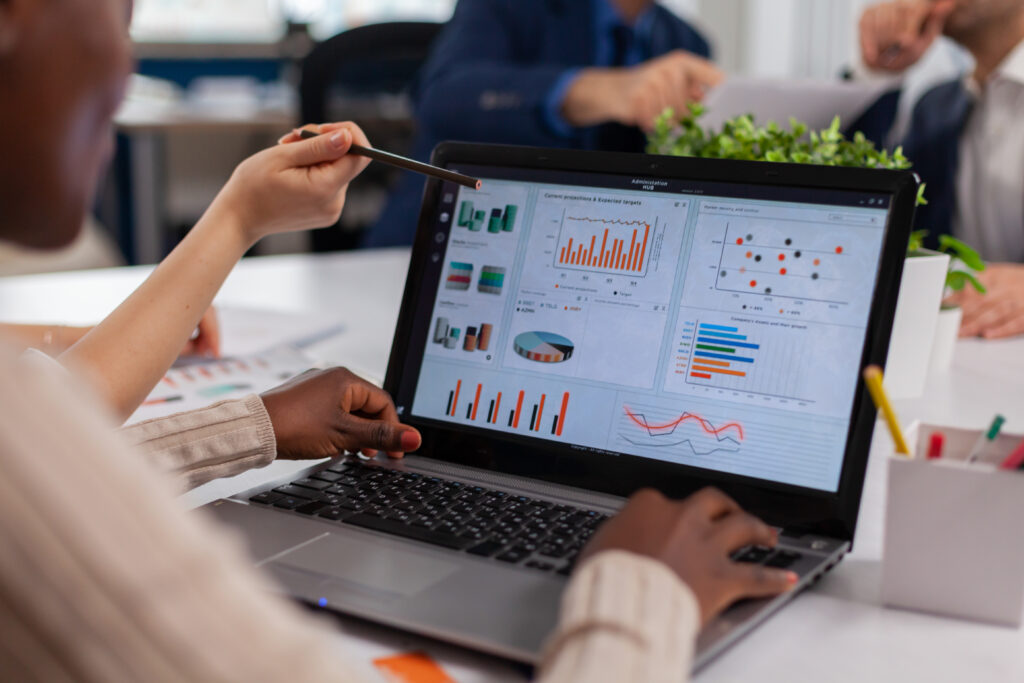
FAQs
- What is algorithmic attribution in advertising?
Algorithmic attribution is an advanced attribution model that uses machine learning to assign value to marketing touchpoints based on their effectiveness in driving conversions. - What’s the difference between rule-based and algorithmic attribution?
Rule-based models use static rules (e.g., first-touch, last-touch) to assign credit, while algorithmic models analyze actual customer behavior to allocate credit dynamically. - How does algorithmic attribution optimize marketing budgets?
By identifying the most impactful touchpoints, algorithmic attribution enables marketers to allocate budgets more effectively, focusing on channels and interactions with the highest ROI.
Ready to take your marketing attribution to the next level? Anderson Collaborative can help you implement data-driven algorithmic attribution models for accurate insights and optimized campaigns. Contact us to start leveraging advanced attribution today.